Artificial Intelligence (AI) is quickly transforming how behavioral health services are delivered and managed. By automating routine tasks, predicting patient behaviors, and delivering data-driven insights, AI is helping clinicians provide more effective and efficient care. In the complex and nuanced field of behavioral health, such tools are indispensable. But not all AI platforms are created equal. As more behavioral health organizations adopt this technology, it’s crucial to understand the key features and capabilities that an effective AI platform should offer.
Unpacking AI: Terminology and Relevance in Behavioral Health
Before we get into the nitty-gritty of software features and functionality, it’s important to understand the language of AI and software-as-a-service (SaaS) platforms.
Understanding these terms gives behavioral health professionals the baseline knowledge they need to ask the right questions and accurately interpret the answers—ultimately helping them select a system that fully aligns with their organization’s clinical needs, operational requirements, and compliance standards.
Here, we break these terms into two categories: AI-specific terminologies and general software/tech terminologies.
AI-Specific Terminologies
- Artificial Intelligence (AI): AI refers to machines or software that mimic human intelligence—learning from experiences, adjusting to new inputs, and performing tasks that would typically require human cognition.
- Augmented Intelligence: This approach to AI emphasizes the technology’s role in enhancing human intelligence, rather than replacing it. Augmented Intelligence is all about machines and humans working together to achieve better outcomes—especially in the context of behavioral healthcare.
- Machine Learning (ML): This component of AI involves using statistical methods to enable machines to improve at tasks as they gain experience performing them.
- Natural Language Processing (NLP): This enables computers to understand and process human language, paving the way for more natural interactions between humans and machines.
- Large Language Models (LLMs): An LLM is a type of AI that analyzes high volumes of data, identifies language patterns and characteristics, and uses that understanding to generate new content in a way that mimics a human.
General Software/Tech Terminologies
- Cloud-Based: Refers to applications or services made available to users on-demand via an internet connection to the cloud computing provider’s servers.
- Privacy: Pertains to the handling and protection of user data to ensure that personal and sensitive information is secured in compliance with applicable laws and regulations.
- Security: Refers to measures taken by a SaaS provider to protect its systems and user data from threats and breaches.
- Encryption: The process of encoding data so that only authorized parties can access it.
- Scalability: Refers to a platform’s ability to handle increased workloads or expand capabilities as the user base or data volume grows.
- Integration: Refers to a platform’s ability to connect and work with other software systems.
- Embedding: This goes a step beyond integration to directly incorporate one application into another, offering a seamless user experience. Whereas integration connects two systems and allows them to communicate with one another, embedding allows users to access the functionality of one system while working inside of another.
- Uptime: The average percentage of time that a platform is operational and accessible to users.
Now that we’re all speaking the same language, let’s dive into the must-have features and characteristics every behavioral health organization should be looking for in an AI solution.
Must-Have #1: AI Built Specifically for Behavioral Health
Behavioral health is a highly specialized field that requires more than just a generalized AI tool. It demands AI models specifically trained in behavioral health language, therapeutic interventions, and the subtleties of patient-provider conversations. Just as a healthcare specialist brings a level of discipline-specific expertise exceeding that of a general practitioner, AI models designed for behavioral health are fine-tuned to pick up on nuances that may go unnoticed by general-purpose models.
Training the Models with Specialized Data Inputs
Think about a typical therapy session. If a client spends 45 minutes talking about their dog, a generic AI model might consider that the focus of the session. However, specialized behavioral health AI could discern that the key therapeutic intervention—or an important patient response—occurred during the remaining 15 minutes, and would focus its analysis on those critical moments instead.
How does an AI model specific to behavioral health achieve this? It all has to do with the data inputs the model is trained on. First, the AI is trained to understand and process language based on general data—think of this as sending the AI to school from kindergarten to 12th grade.
After that, it goes to “graduate school,” where it is fine-tuned using behavioral health-specific data. During this process, it learns to not only understand the clinical language used in behavioral health practice, but also pick up on other relevant conversational elements that may arise in a session.
Fine-tuning the Models with Specialized Clinical Experts
To ensure the model’s effectiveness, it’s critical that this process of fine-tuning and validation be conducted by a skilled clinical team who understand contextual subtleties and can accurately evaluate the results generated by the AI. Furthermore, the testing and research the team carries out to prove that the model works should be rigorous, transparent, and grounded in scientific evidence.
“For any technology that leverages AI models, effectively training the models to perform with a high degree of accuracy is critical,” Shiri Sadeh-Sharvit, PhD, Chief Clinical Officer at Eleos Health, explained in this article. “For a model specific to behavioral health, that level of training is only possible with input from clinical experts—which is why our clinical team is a core differentiator for us at Eleos. Furthermore, the overall effectiveness of the model hinges on the quality of the data used to train it. By training our models with real-world session data, we enhance their applicability in the intended context: community-based behavioral health clinics.”
Given the importance of specificity, here are a couple of key questions to ask when considering an AI platform for behavioral health:
- Is the AI model trained on a data set specific to behavioral health?
- What clinical experts are involved in testing and research efforts to validate the effectiveness of the AI model?
A behavioral health-focused AI model goes beyond simply processing language—it deciphers the complex layers of therapeutic conversations, honing in on what truly matters in the session. By ensuring your AI platform has this level of specialization, you can significantly enhance the quality and effectiveness of the platform’s output—and consequently, your care.
Must-Have #2: Seamless Alignment with Existing Tech and Workflows
It doesn’t matter how impressive a tool is if clinicians can’t easily use it. That’s why an AI vendor’s understanding of provider workflows is crucial to successfully integrating their solution in your organization with as little disruption as possible. It’s important that software providers map out workflows, study clinician-client interactions, and adapt their AI solutions accordingly. The goal is to make sure the AI tool adds value without causing a hassle.
To that end, here are a few factors to consider.
Interface and Usability
Change can be challenging, even when it brings benefits. On top of seamlessly integrating into provider workflows, behavioral health AI also must be user-friendly. An intuitive interface with a short learning curve will encourage quicker adoption.
Minimal IT Requirements
Another challenge to consider is the work required of your organization’s IT team during the implementation phase. With an ideal AI solution, your IT team’s lift will be minimal—allowing the software to offer value right away.
Compatibility with EHR and Telehealth Tools
Adopting AI shouldn’t require a total overhaul of your existing tech stack. Whatever solution you choose needs to work with the technology you already have—including your EHR and telehealth tools. Compatibility is key, and it’s important to understand whether the AI solution integrates or embeds with your existing technology—and how the connection works.
Integration vs. Embedding
There are two ways a new software can interact with your current software: integration and embedding.
Integration is the traditional way that software platforms “talk” to each other. Integration requires engineers on both sides to grant permissions and update code. Tech companies commonly charge for integrations and take extra time to get them done.
For example, you might want to purchase a new teletherapy platform. But in order for that software to connect with all your current solutions, you’ll typically need to contact your other vendors and ask them to make the integration happen. These companies might charge substantial fees and take months to finish the process—meaning you’ll have a new platform that you can’t fully leverage until every team finishes their part of the integration process.
Embedding is a new, more versatile process for connecting software systems. When a software can “embed” within other systems, the vendor doesn’t have to request permission or access codes to begin working with the other software. The new technology can work alongside your existing systems immediately—so you don’t have to wait (or pay) for another team’s work before you begin implementation. A common way for web-based technologies to embed with each other is through a browser extension.
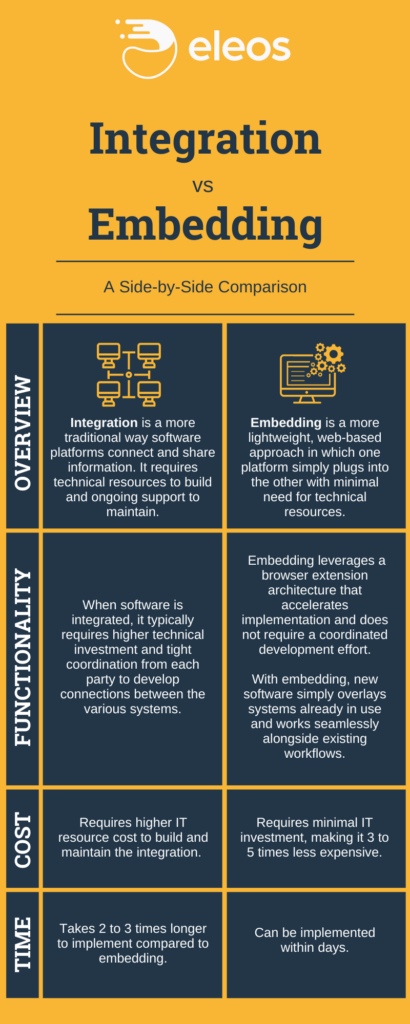
Considering these factors, here are a few more questions to ask prospective healthcare AI vendors:
- Does the AI solution fit into current provider workflows?
- Does the solution work for both in-person and telehealth sessions?
- How much IT work is required to implement the solution?
- How does the solution interact with existing technology, such as an EHR? Does your product work with my current technology? If so, does it use integration or embedding?
With a well-rounded understanding of these factors, behavioral health organizations can seamlessly incorporate AI tools into their workflows—adding value and enhancing clinician productivity without causing friction.
Must-Have #3: Comprehensive Support from the AI Vendor
Implementing an AI solution isn’t a one-time transaction; it’s a journey requiring a collaborative, continuous partnership with the AI vendor. The vendor should provide a comprehensive support framework, including scaled implementation plans, training, ongoing support, and maintenance.
Here are some specific things to look for.
Scaled Implementation Plan
An effective vendor will provide a phased or scaled implementation plan, easing the transition to the new system. That way, you can account for your organization’s specific needs without overwhelming staff or disrupting existing processes.
Training and Ongoing Support
Technology can’t help you if you don’t know how to use it. That’s why your AI vendor should assist with training and provide comprehensive instructions to support the onboarding process. The vendor should also offer ongoing support, addressing any technical issues promptly and effectively.
Continual Measurement and Improvement
Post-implementation, it’s crucial to assess the AI solution’s performance on an ongoing basis. The vendor should support this evaluation process, providing relevant data and engaging in conversations about the solution’s impact, areas for improvement, and strategies for optimizing results.
Transparent Communication
The vendor should be transparent about their product roadmap, involving users in discussions about potential new features and improvements. This transparency can help build a stronger partnership and ensure that the solution continues to meet your organization’s needs over time.
Dedicated Point of Contact
Clear and smooth communication is key. A dedicated point of contact at the vendor—such as a customer success manager—can help streamline communication, making it easier to address concerns, clarify doubts, and facilitate a more personalized service.
Here are some suggested questions to help you dig deeper into the level of service you can expect from an AI vendor:
- What support do you provide once a customer signs on with you?
- What do you guarantee to every customer?
- How long does it typically take to fully implement your AI solution? What happens if you can’t meet the timeline we agree on?
- Can you provide us with a scaled implementation plan?
- Are there training requirements for this implementation, and will the vendor assist with this? Does the vendor provide ongoing support and maintenance, and how?
- Who will be our primary point of contact at your company? How quickly can we expect them to respond?
When you receive high-quality support from your AI vendor, your behavioral health organization can better manage the transition to using the AI solution consistently—ultimately maximizing its benefits with minimal disruption to existing workflows.
Must-Have #4: Value and Performance Insights
Implementing AI technology in behavioral health settings is not just about adding another tool to your tech stack. It’s about driving measurable value for your organization—including enhanced quality of care and improved overall effectiveness.
A worthwhile AI vendor should be able to clearly articulate their value proposition. They should explain how their technology will benefit your organization, and how they’ll partner with you to measure the impact.
Here are a few measurement approaches to explore as you assess each AI platform.
Productivity and Efficiency Metrics
AI can provide insights into provider productivity with indicators such as the time it takes for a provider to write and finalize the progress note after each session. With AI, these times should decrease significantly, allowing providers to focus more on patient care. Proxy metrics—such as the number of patients a provider sees each day before and after AI implementation—can also highlight efficiency gains.
Clinical Outcome Metrics
AI’s impact on clinical outcomes is another important area to measure. When providers spend less time on documentation, they can focus more on patients, leading to improved treatment outcomes. Indicators to watch include patient satisfaction scores, symptom improvement, and session attendance rates—all of which should increase with an effective AI solution.
Staff Satisfaction Metrics
Finally, consider the impact of AI on staff satisfaction, engagement, and stress levels. Given the high turnover rate in behavioral health—largely due to burdensome documentation requirements—AI should ideally enhance job satisfaction. Measuring the net promoter score (NPS) of clinicians using the AI tool can provide insights into their satisfaction levels. Over time, you might also expect to see higher staff retention rates.
In light of these considerations, here are some questions you should ask the AI vendors you are evaluating:
- What value do you expect your technology to provide to our organization?
- How can we measure the value your technology is delivering?
- What metrics or KPIs should we be tracking?
- How do you facilitate ongoing value evaluation and monitoring?
The right AI solution doesn’t just automate administrative tasks—it offers strategic insights to help you continually optimize performance, improve care quality, and boost staff satisfaction. But to properly measure the success your vendor delivers in those areas, they must set clear expectations upfront.
Must-Have #5: Data Security and HIPAA Compliance
In the healthcare world, data security is not just a preference—it’s a necessity. Ensuring data safety and maintaining compliance with the Health Insurance Portability and Accountability Act (HIPAA) are non-negotiables, as data security breaches or non-compliance can result in significant legal and financial consequences. AI vendors play a critical role in fulfilling these obligations.
Navigating the Challenges of Data Security and HIPAA Compliance
The implementation of healthcare AI technology introduces a variety of data security and HIPAA compliance challenges. First, the vendor must have appropriate certifications. Look for those that are compliant with HIPAA and other relevant security standards, such as SOC 2. These certifications provide assurance that the vendor is adhering to best practices.
Focusing on Audit Controls, Privacy Controls, and Security Controls
When evaluating an AI vendor’s data security and compliance practices, focus on three areas:
- The first is audit controls, which include conducting annual risk assessments.
- The second is privacy controls, which foster limited data access according to the principle of least privilege (a computer security concept in which a user is given the minimum level of access necessary to complete their job functions).
- The third is security controls, including the implementation of tools to secure endpoints (any device that can connect to a network is considered an endpoint) and APIs (Application Programming Interfaces, a.k.a. sets of rules that allow different systems to connect with each other). Furthermore, make sure the vendor has a plan in place for cross-region disaster recovery (a strategy that involves replicating and hosting data and applications in more than one geographic location to ensure availability during a disaster).
Understanding Privacy and Security
It’s also important to understand the difference between data privacy and data security. While security focuses on protecting data from external threats, privacy involves controlling who has access to data within the organization. Both are critical to maintaining HIPAA compliance and keeping patient information safe.
Mitigating Risks with Third-Party Applications
In cases where the AI platform is supported by third-party applications, there is an added layer of complexity in ensuring data security and compliance. It’s essential to understand how these third-party providers handle data and what safeguards they have in place.
When vetting an AI vendor for your behavioral health organization, ask the following questions:
- How do you ensure data security and maintain HIPAA compliance?
- What security certifications do you hold (e.g., HIPAA, SOC 2 with HITRUST compliance)?
- How do you handle audit controls, privacy controls, and security controls?
- In the event of a data breach or disaster, what are your recovery measures?
- If your AI is supported by third-party applications, how do you ensure these applications maintain the same level of security and compliance that you do?
Choosing an AI vendor that prioritizes data security and HIPAA compliance is a must. Their ability to navigate the complexities of data protection can greatly impact your organization’s risk exposure and compliance status.
Must-Have #6: Customization and Adaptability
Each behavioral health organization has unique needs, which means a “one-size-fits-all” AI solution probably won’t cut it. AI solutions must be customizable based on individual needs, and they must be flexible enough to adapt and evolve in line with future technologies.
Features Tailored to Your Needs
You should be able to tailor your AI solutions to fit the specific needs and workflows of your organization. This customization could come in the form of special data-reporting setup, functionality to suit specific user roles, or even integrations with existing systems. Vendors should work with you to understand your unique requirements and adapt their solution in a way that enables your organization to improve operational efficiency and clinical outcomes.
Adaptability to Existing and Future Technologies
Technology solutions should not only integrate seamlessly with your existing technologies, but also be ready to adapt to future ones. The behavioral health landscape is changing quickly, and the last thing you want is to be left behind with an outdated tool. An adaptable AI solution is designed with future growth in mind, ensuring it can integrate with new technologies as they emerge.
Feedback-Driven Development
Beyond prebuilt customizations, the best software providers take into account client feedback as they plan out their product and feature roadmaps. They view their customer relationships as partnerships, continuously demonstrating how they’ve made adjustments or added features based on client needs and suggestions. This feedback loop ensures the solution continues to evolve alongside the changing needs of your organization. A forward-thinking tech vendor doesn’t just react to feedback; they proactively engage with you and other customers to understand your experiences, identify potential improvement areas, and forecast future needs.
To make sure a potential solution is flexible enough to add value for years to come, behavioral health leaders should ask:
- How can the AI solution be customized to fit our unique needs and workflows?
- How do you collect and incorporate user feedback as part of your development process? Can you give examples of changes you’ve made based on client feedback?
- Do you have a process in place to create your product and feature roadmap based on user needs and technology advancements?
An AI solution’s ability to be customized and adapted will ultimately determine how useful and long-lasting it is. You need a vendor who can provide this flexibility while still maintaining a high level of usability and support.
Must-Have #7: Clinician-Centered AI Documentation
In the healthcare community, there’s a valid concern about overreliance on AI. The human touch, combined with years of training and provider intuition, plays an irreplaceable role in effective care delivery. Emotions and subtle cues are important when it comes to behavioral health.
AI offers the ability to sort through data, streamline tasks, and predict outcomes. However, it cannot fully grasp the weight of a silent pause, a shift in tone, or the meaning behind unspoken words. Clinicians bring with them a wealth of experience and understanding that AI cannot replicate.
So, what constitutes an effective AI-driven documentation tool?
Provider Review and Oversight
The AI system might make suggestions based on patterns and data, but clinicians should review and approve each statement to ensure accuracy and relevance.
Iterative Feedback Mechanism
An effective AI system should be adaptive, refining its documentation suggestions based on continuous clinician feedback—including which AI-generated suggestions are (and are not) accepted as well as what edits the clinician makes to the automated note content.
AI as Support, Not Replacement
The AI’s primary role should be to assist (i.e., by providing preliminary drafts or highlighting patterns). The final note should, without a doubt, reflect the clinician’s insights.
Notes Unique to Each Client
Some documentation tools use “dot phrasing,” a digital shorthand that expands keywords into phrases. While efficient, dot phrasing is limited to predefined templates and cannot adapt to specific sessions or offer personalized clinical insights. Case-specific content is crucial to ensuring that nuanced and individual needs are captured accurately in documentation.
When evaluating an AI solution, ask:
- Does the system ensure that the clinician remains the principal authority in the documentation process?
- Is there a robust mechanism for the AI to evolve based on clinician feedback?
- Does the system require clinician approval before documentation is finalized? Is the software structured for passive clinician oversight, or does it demand active involvement (i.e., inputting key phrases or selecting from a list of options)?
- Does the system generate note content based on individual case details as opposed to generic predefined options?
In behavioral health documentation, the AI should serve as a supportive tool, with the clinician directing the process based on their unmatched expertise.
Evaluating Risks and Impact: Balancing Value and Potential Challenges
When considering the implementation of AI technology in your organization, a balanced perspective is vital. Here’s a comparison of potential risks and rewards to help you better evaluate the decision.
Cost vs. Return
Initial implementation and ongoing operation of AI technology will require a financial investment. However, the potential return could far outweigh these costs through improved efficiency, reduced manual labor, and increased quality of care.
Learn more about the financial benefits of behavioral health AI (and calculate your organization’s potential ROI) here.
Time Spent Implementing vs. Time Saved
Implementation will require time for system integration and staff training. But once in place, the AI platform can streamline many administrative tasks, free up staff time, and speed up workflows—delivering substantial time savings in the long run.
Data Security Risks vs. Enhanced Compliance
While the use of AI technology introduces potential data security risks, a robust AI platform can enhance HIPAA compliance and provide stronger data security measures than manual note-taking methods.
Complexity of Integration vs. Streamlined Workflows
Although integrating AI with existing systems may require time and effort, once the technology is up and running, AI can greatly streamline workflows, reduce errors, and improve the overall quality of service.
The decision not to implement AI technology presents its own risks. In an increasingly digital world, falling behind the tech adoption curve can lead to decreased efficiency, missed opportunities for improved care, and a competitive disadvantage. As Josh Cantwell, COO of GRAND Mental Health, explains in this article, “[AI] is not the future; this is the present. This isn’t even us being ahead. This is one of those things that’s just keeping us level with the rest of the playing field.”
Selecting the Right AI Platform for Your Behavioral Health Organization
Choosing the right AI platform is critical. Having the right combination of features and capabilities at your disposal will weigh heavily on your organization’s ability to deliver high-quality care, analyze performance, and achieve goals. Reflect on the following must-haves when making your decision:
- AI Built Specifically for Behavioral Health
- Seamless Alignment with Existing Tech and Workflows
- Comprehensive Support from the AI Vendor
- Value and Performance Insights
- Data Security and HIPAA Compliance
- Customization and Adaptability
- Clinician-Centered AI Documentation
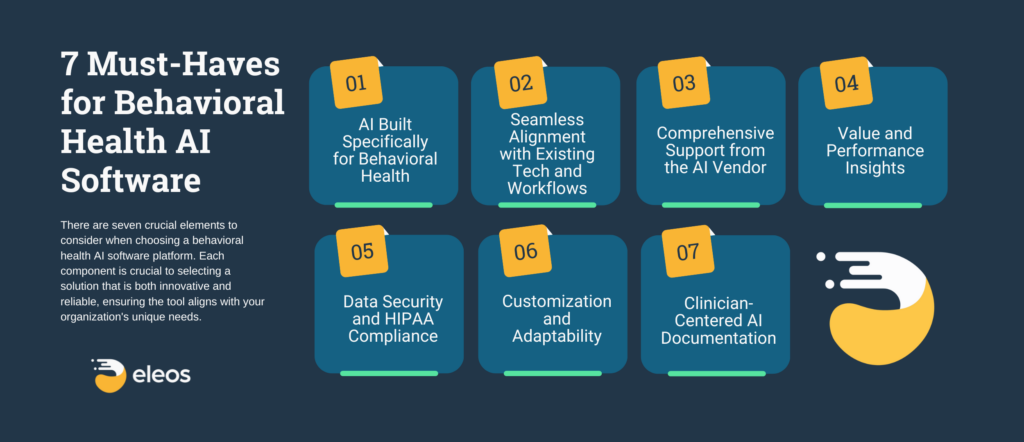
The right tech solution will excel in all of these areas, providing benefits and value across your entire organization.
Eleos Health’s CareOps Automation platform delivers all of the above and more. Eleos offers a robust documentation automation and quality feedback solution that values user needs, prioritizes data security, and is dedicated to continuous improvement. Not only does Eleos provide valuable performance insights, but the platform also offers a myriad of customization options and seamlessly embeds into existing technologies, including virtually any EHR.
Remember, the right choice will position your behavioral health organization for operational and clinical success for years to come. The stakes are high, so take the time to consider all your options. The right software is a game-changer—it can transform your organization, making care delivery a smoother, more enjoyable and effective process for clients and providers alike.