Today’s world is full of technology that can think and learn. It’s helping us in our homes, in our workplaces, and even in the way we care for our health. And for providers in the behavioral health field, these technologies are becoming important tools for delivering the best possible care.
It’s becoming increasingly clear that the highest-quality mental healthcare happens when technology and humans work together. Technology can handle tasks that are time-consuming or repetitive for humans—like tracking patterns, crunching numbers, and analyzing data.
As tech takes on these to-dos, humans are free to connect with people on a personal level, provide empathy, and use intuition to guide care. This is the partnership innovative behavioral health providers strive for: leveraging technology to enhance human skills—not replace them.
That’s the key difference between Artificial Intelligence and what the healthcare industry calls “Augmented Intelligence”: one aims to make humans redundant, while the other aims to make them more effective.
In this guide, we’ll explore the inner workings of Artificial Intelligence and Augmented Intelligence, address common misconceptions about healthcare AI, and illustrate the value of using these technologies in behavioral health practice.
Understanding Artificial Intelligence
What is Artificial Intelligence?
In its simplest form, Artificial Intelligence (AI) refers to the capability of a machine or a computer program to mimic and execute tasks that typically require human intelligence. This could include understanding natural language, recognizing patterns, solving problems, and learning from experiences.
“AI is the capacity of a machine to reproduce a task that would usually require human intelligence to achieve.”
-Samuel Jefroykin, Director of Data & AI Research, Eleos
The term “artificial” denotes that this form of intelligence is created by humans using complex algorithms and data. It’s not an organic or naturally occurring phenomenon. The “intelligence” part of AI signifies the ability of these systems to understand, learn, and apply this learning to achieve specific goals.
How does AI work?
AI systems are intricately designed and trained to perform specific tasks, and through a process called Machine Learning (ML), they constantly learn and refine their abilities over time.
Unlike humans, AI doesn’t forget. It doesn’t make the same mistake twice, and with its ability to process vast amounts of data at breakneck speed, it learns and improves at a pace that’s astronomically faster than ours.
Let’s dive deeper into the ML process:
- Data Collection: Every AI journey begins with data. This data, which could range from text and images to numeric inputs, forms the foundation for the task the AI is designed to perform.
- Data Cleaning: Once the data is collected, it must be cleaned. This involves eradicating errors, filling in missing values, ensuring consistency, etc.
- Data Transformation: Next, the cleaned data is transformed into a format that the AI can process, which often means converting it into numbers or other mathematical values.
- Model Training: The AI uses this transformed data to learn how to perform its assigned task. It isn’t given any specific rules or guidelines; rather, the AI learns autonomously from the data it has been provided.
- Model Testing and Feedback: After training, the AI is put to the test. It applies what it has learned to perform tasks and make predictions. The AI’s outcomes are then compared to actual results, and feedback is provided. This allows the AI to correct any errors, adjust its parameters, and further refine its understanding.
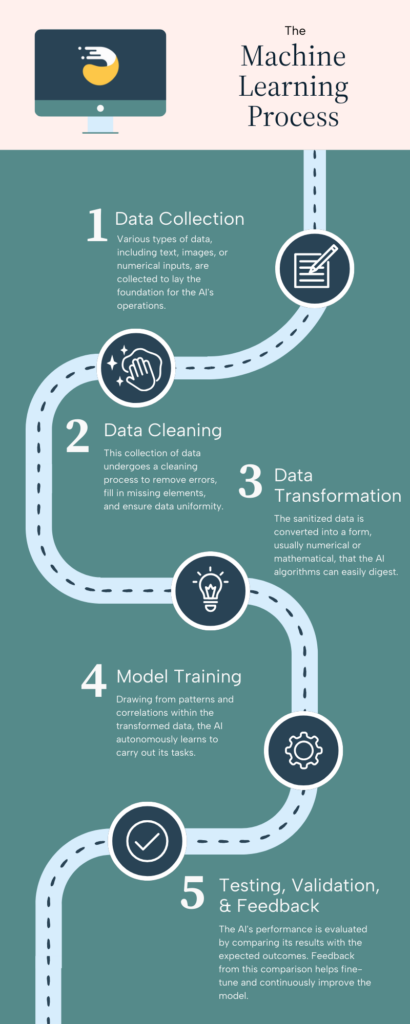
Consider an AI designed to predict house prices based on location, size, and rent values. It is first given a dataset of houses with these attributes and their actual prices. From there, it embarks on a pattern-finding quest as it reviews the data, with a goal of predicting house prices as accurately as possible.
Crucially, the AI isn’t making assumptions or following any pre-programmed instructions. It’s not calculating a value based on a rule like, “If the house is in X location and has Y square footage, then it should cost Z dollars.” Instead, the AI is identifying patterns in the data and using these patterns to generate predictions—a process driven solely by the data itself.
In short, AI is in a continuous loop. It starts with data, learns from this data, applies its learnings in order to perform tasks, receives feedback, and uses that feedback to refine its approach. With each iteration, the AI grows smarter and more efficient, continuously improving its ability to accomplish the task at hand.
Listen to our AI 101 podcast episode to learn more about the inner workings of behavioral health-specific artificial intelligence.
What are the building blocks of AI?
If you think of AI as an intricate structure, the building blocks of that structure are the data and algorithms that power it. These components enable AI to learn, think, and act in ways that seem intelligent. Here’s an overview of these building blocks:
- Data: This is the raw material driving any AI model. The quality and quantity of data that an AI model has access to directly influences its performance. This could be anything from numeric data and text to images and sound. The more diverse and comprehensive the data, the better the AI’s ability to understand, learn, and make accurate predictions.
- Algorithms: An algorithm is like a recipe for the AI—it outlines the steps needed for the AI to learn from the data and make predictions or decisions based on that learning. Machine learning algorithms are the heart of AI, enabling it to learn autonomously from the data provided.
- Model: The model is the representation of what the AI has learned from the data. It’s a mathematical equation that the AI uses to make predictions or decisions. The model evolves and improves as the AI learns more.
- Training: This is the process by which an AI learns from the data. The AI is fed the data and uses its algorithms to analyze it, find patterns, and build a model. This process can involve supervised learning (where the AI is given both the inputs and the desired outputs), unsupervised learning (where the AI is given only the inputs), or a combination of both.
- Testing and Validation: Once the AI has been trained and a model has been built, that model is put to the test. The AI uses the model to make predictions or decisions based on new data, and these results are then compared to actual outcomes to determine how accurate the AI is.
- Feedback and Optimization: After testing, the AI receives feedback on its performance. This feedback is then used to fine-tune the model, helping the AI to learn from its mistakes and improve its future performance.
When we piece together these building blocks—data, algorithms, models, training, testing, and feedback—we get a complex, intelligent system that can learn from experience, adapt to new situations, and make informed decisions or predictions.
The power of AI lies not just in its ability to mimic human intelligence, but in its potential to exceed it. By analyzing and learning from vast quantities of data at a speed and scale that’s beyond human capacity, AI can uncover insights, identify patterns, and make predictions that are often more accurate than those made by humans.
How does AI know what information to use when formulating a response?
AI isn’t just guessing when it generates responses; it’s retrieving and prioritizing information based on context. In behavioral health, this means AI pulls the most relevant data points from past sessions, clinical guidelines, and evidence-based treatment models instead of relying on static, pre-trained decision mechanisms.
To do this, advanced AI systems use a method called Retrieval Augmented Generation (RAG). Instead of only using information that was originally trained into the model, RAG enables AI to search a structured knowledge base in real time, helping ensure responses are grounded in relevant, accurate, up-to-date information.
But how does the AI find the best information quickly?
Think of a traditional AI database as a box of unorganized papers. If you needed to find information specifically about anxiety, you’d have to dig through documents one by one until you spotted the word. This process is slow, and there’s no guarantee you’ll find the most relevant information first.
Most advanced AI systems are now using Vector Databases, combined with RAG, to improve recall. Vector Databases (also known as Vector DB) work more like a well-organized filing cabinet. Instead of scanning randomly, the information is sorted into “files” by meaning, and those files are then numbered. The AI then uses those numbers to pull the information most relevant to the current request.
For example, let’s say a therapist working with a particular client asks the AI for insight on the client’s progress. The AI doesn’t just pull any old notes—it searches the Vector Database to find:
- The most relevant past therapy notes for that client.
- Similar cases, which it then uses to identify effective interventions.
- Best practices from evidence-based care models.
By combining real-time data retrieval through RAG with the structured, meaning-based search capabilities of Vector DB, advanced behavioral health AI tools help therapists get the right information, at the right time. Instead of making assumptions or sifting through irrelevant data, this type of AI empowers clinicians to make faster, more informed decisions without the guesswork.
Exploring Augmented Intelligence
What is Augmented Intelligence, and how is it different from Artificial Intelligence?
Augmented Intelligence represents a significant shift in how we perceive and use the power of AI. The concept of Augmented Intelligence moves away from the idea of AI as an autonomous entity that replaces human intelligence—and toward a view in which AI acts as an auxiliary force that enhances human thinking and reasoning. This flavor of AI is preferred in most clinical communities, because it emphasizes the importance of the human who is using the technology.
The American Medical Association (AMA) describes Augmented Intelligence as a conceptualization of Artificial Intelligence that emphasizes AI’s assistive role. In essence, it is about using AI to amplify human intelligence rather than rendering it obsolete.
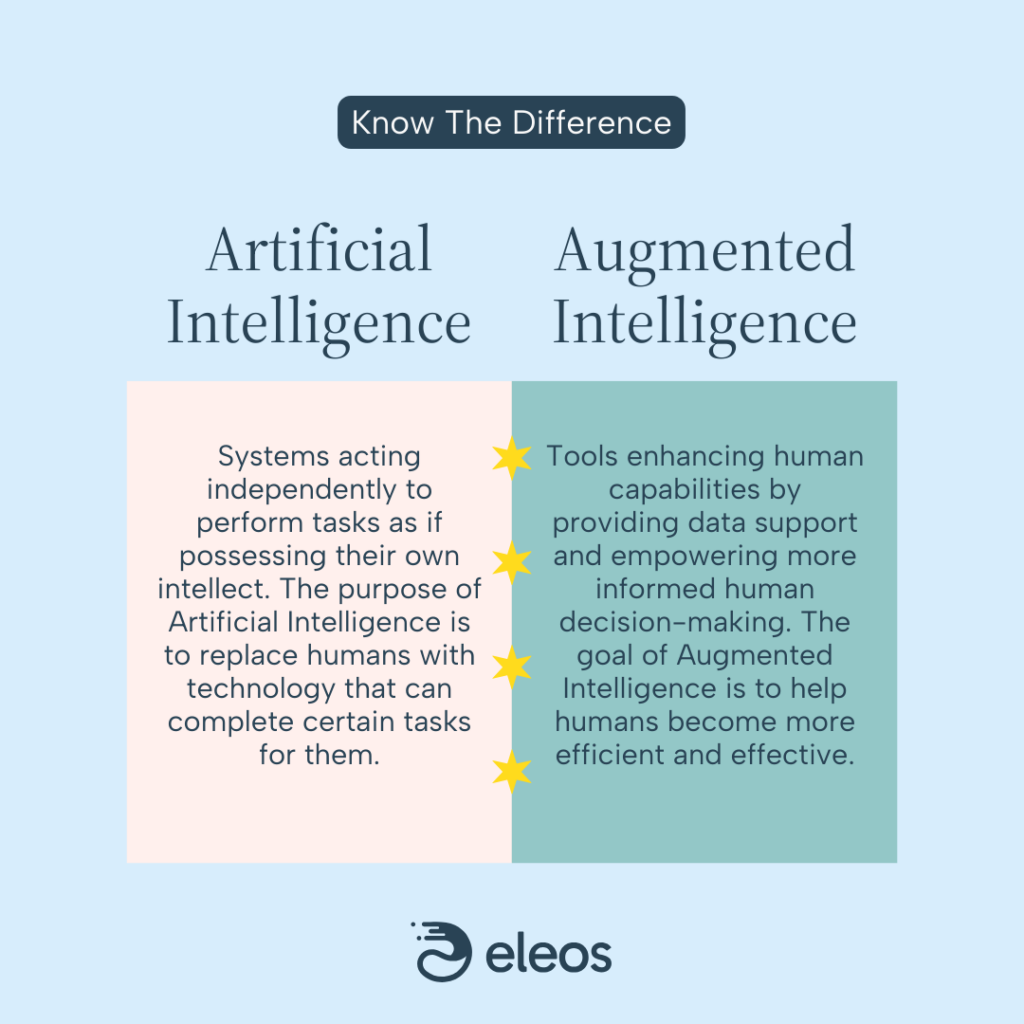
Samuel Jefroykin, Director of Data & AI Research at Eleos, uses this metaphor to explain the difference between Augmented Intelligence and Artificial Intelligence: Augmented Intelligence is like a calculator aiding a mathematician. The calculator, while capable of performing complex calculations swiftly and accurately, doesn’t eliminate the need for a mathematician. Instead, it enables the mathematician to work more efficiently and focus on higher-level problem-solving. The calculator augments the mathematician’s intelligence—but doesn’t replace it.
In a similar vein, Augmented Intelligence serves as a powerful tool in the hands of clinicians. Instead of replacing clinicians, it augments their ability to make informed decisions, recognize patterns, and provide effective care. Augmented Intelligence serves to strengthen the clinician’s expertise and intuition, enriching the decision-making process with data-driven insights that the clinician likely could not produce on their own.
How are Augmented Intelligence models created?
The creation of Augmented Intelligence models is a detailed process that, although similar to traditional AI training, aims to provide distinct, clinically relevant output. Eleos’ AI is trained daily by doctoral and masters-level providers with expertise in Evidence-Based Treatments (EBT). Using their clinical insight, these providers guide the AI’s training, setting benchmarks based on important metrics like recall (the AI’s ability not to miss the target it’s asked to identify) and precision (the AI’s ability not to falsely identify other items as the target).
The experts train the AI to correctly discern the presence or absence of clinically pertinent content. For example, they evaluate whether a statement involves a mood check or correct use of mindfulness. Defining what is “good enough”— for example, by establishing acceptable Type I (the mistake of saying something has happened when it hasn’t) and Type II (the mistake of saying nothing has happened when it has) error rates—falls under the purview of Eleos’ clinical analysts and data scientists.
This process, similar to standard psychometric methods for determining valid reliability levels in psychological tests, is repeated thousands of times across every variable tracked by Eleos, ensuring the system accurately distinguishes between relevant and irrelevant provider information.
As Jefroykin highlights, the distinction between Augmented Intelligence and traditional AI lies in the design of the outputs and the way data is presented to the user. It’s about constructing models that offer user-friendly, intuitive suggestions to the clinician.
Furthermore, Jefroykin makes a clear distinction between a general AI model and one focused on behavioral health. While a general model summarizes the content of a conversation, a behavioral health model delves into the clinical aspects. For instance, it is trained to understand the therapeutic interventions running under the surface during a conversation. This involves initially training the AI on general data and then fine-tuning it with behavioral health data.
In essence, building a behavioral health Augmented Intelligence model means equipping the AI with a foundational understanding (akin to K-12 education), and then sending it to “grad school” for a behavioral health specialization. The end goal is an AI that’s not only proficient in behavioral language, but also capable of processing any subject that might surface during a session. Most importantly, it prioritizes delivering the insights the clinician needs in order to make informed decisions—not prescribing what the clinician should do.
Learn more about the importance of using AI built specifically for behavioral health.
How is Augmented Intelligence used in clinical practice?
In real-world clinical settings, therapists use Augmented Intelligence to deliver better, more personalized care. By analyzing variables such as a patient’s history, symptoms, treatment responses, and behavioral patterns, Augmented Intelligence can generate suggestions for tailored therapeutic interventions. This empowers therapists to concentrate on implementing these strategies and fostering strong patient relationships.
But beyond clinical support, Augmented Intelligence is also playing an increasingly important role in organizational compliance and risk management—further enabling staff to stay focused on care. Tools like Eleos Compliance assist providers by automatically checking notes for quality and accuracy, ensuring that interventions, progress updates, and action plans are properly documented. In essence, Augmented Intelligence acts as a backup safeguard, catching potential compliance issues before they become costly downstream problems.
“If you give a mathematician a calculator, you just help them save thousands of hours calculating. In the end, they are not less of a mathematician just because the calculator is faster. It’s the same when you give AI tools to a clinician: you augment their abilities. That’s the power of human intelligence plus AI.”
-Samuel Jefroykin, Director of Data & AI Research, Eleos
What sets Augmented Intelligence apart is its capacity for continuous learning and adaptation. The system consistently refines its algorithms based on data processed and feedback received, enhancing the precision and effectiveness of future recommendations. As therapists interact more with the system, it becomes an increasingly invaluable tool, facilitating more accurate decision-making and contributing to even greater patient outcomes improvement over time.
Jefroykin says one example of this is the platform’s autocomplete feature, which auto-adjusts weekly based on each therapist’s unique writing patterns. So, each week that the therapist uses Eleos, the system gets better and better at writing notes in that therapist’s style.
In addition to building in autonomous learning, Eleos leverages human reviewers to investigate subsets of data where the AI’s suggestions weren’t adopted by the user. These experts can then adjust the models based on their findings, further refining the algorithms and helping the AI system continually adapt to better assist its users over time.
Scientific Validation: The Evidence Supporting AI and Augmented Intelligence Models
AI and Augmented Intelligence have shown promising applications in the field of behavioral health, as demonstrated by a growing body of scientific evidence:
- Effective Integration in Behavioral Health: AI and Augmented Intelligence are beneficial when incorporated into regular mental health care practices. For instance, Eleos has been shown to enhance the process of measuring treatment effects in cognitive-behavioral treatment for depression and anxiety. The tool non-intrusively gathers essential data, thereby providing a comprehensive view of the patient’s progress and aiding clinical decision-making.
- Accuracy in Therapy Components Detection: Machine learning models have been shown to accurately identify components of therapy sessions, such as the assignment of therapeutic homework. This capability can provide valuable insights into treatment sessions, offering a more objective understanding of therapy dynamics and adherence to evidence-based treatment protocols.
- Facilitation of Better Clinical Outcomes: When implemented in behavioral health settings, AI platforms can facilitate better clinical outcomes for patients. A randomized controlled trial (RCT) showed that an AI platform improved symptom reduction and patient retention rates in outpatient therapy for individuals with depression and anxiety disorders. These results highlight the potential for AI to enhance the delivery and effectiveness of behavioral health interventions.
These studies validate the usefulness of AI and Augmented Intelligence models in behavioral health care. They demonstrate how these technologies can provide insights into therapy dynamics, enhance the delivery of care, and improve patient outcomes.
The Case for Augmented Intelligence in Behavioral Health
As with any industry, change within healthcare—particularly within behavioral health—is often slow and met with resistance. However, the potential benefits of Augmented Intelligence are significant, and it’s worth taking on the challenges of integrating these systems.
Why should behavioral health providers use Augmented Intelligence?
Behavioral health is uniquely human-centric, as it is rooted in the complexities of human emotions, behavior, and cognition. Here, clinicians are not just dealing with physical tests or symptoms; they are analyzing human experiences that are often nuanced and variable. Despite significant advancements in AI, no machine or algorithm can fully understand these complexities the same way a human clinician can. The empathy, insights, and personal touch offered by a clinician remain irreplaceable, making them crucial to effective therapy.
In recognition of this, the design philosophy of Augmented Intelligence is not about replacing the clinician; it’s about enhancing their capabilities. Augmented Intelligence is designed to work alongside clinicians, equipping them with sophisticated tools and data-driven insights so they can offer more personalized care. By acknowledging the nuances of human behavior, Augmented Intelligence ensures that the therapist remains at the helm of decision-making.
What are the benefits of Augmented Intelligence in behavioral health?
Augmented Intelligence offers a multitude of benefits in the realm of behavioral health, including:
- More Personalized Care: The use of Augmented Intelligence in behavioral health not only streamlines patient care, but also personalizes it. In addition to serving up insights specific to individual patients to help guide customized care paths, AI helps providers maintain more comprehensive documentation of each patient’s history. When providers can easily access important details about patient history, they can deliver more personalized, trauma-informed care—resulting in a higher-quality experience for the patient.
- Greater Efficiency: By automating mundane administrative tasks like documentation and data entry, Augmented Intelligence frees up more time for therapists to interact with their patients. Studies suggest that clinicians spend nearly half their day on data entry and other computer-based administrative tasks. By cutting down this time, Augmented Intelligence can significantly increase organizational efficiency. The Eleos platform, for instance, is shown to reduce the time providers spend on clinical documentation by 70% or more.
- Improved Decision-making: Augmented Intelligence offers data-driven insights that aid in clinical decision-making. By analyzing various data points such as patient history, symptoms, and treatment responses, it can provide clinicians with valuable insights, guiding them toward more accurate diagnoses and treatment decisions. For example, a study funded by the National Institute for Mental Health found that a machine-learning algorithm was able to predict with 80% accuracy whether a person would develop psychosis based on their speech patterns—a full two years before doctors made such a diagnosis. Such predictive capabilities can greatly aid clinicians in their treatment process.
- More Targeted Feedback: Just like humans, Augmented Intelligence systems have the ability to learn and adapt over time. The algorithms refine their accuracy and efficiency based on the data they process and the user feedback they receive. This means that the more the system is used, the more accurate it becomes, thereby continually improving its recommendations. In this way, Augmented Intelligence can facilitate better patient outcomes. It also enables care providers to continually refine their skills and techniques based on the insights provided. For instance, the AI model developed by Eleos not only provides insight into client care, but also generates feedback on the provider’s clinical techniques, enabling them to learn and improve over time.
- Stronger Compliance & Risk Prevention: AI tools like Eleos Compliance proactively review every note for missing information, accuracy issues, and adherence to the golden thread. This helps organizations reduce audit risk, insurance denials, and regulatory penalties—without requiring additional staff time.
- More Robust Clinical Supervision & Quality Improvement: AI is now enhancing clinical oversight by providing objective session insights that help supervisors guide and support their teams. Eleos provides data analysis on key data points such as therapist talk-to-listen ratios, intervention usage, and treatment fidelity. Providers can then use this information to uplevel themselves—and supervisors can use it to drive more thoughtful and meaningful coaching discussions.
What are some limitations of Augmented Intelligence in behavioral health?
Despite the numerous benefits, there are some limitations to bear in mind as organizations implement Augmented Intelligence support:
- Dependency: Over-reliance on the system may limit the clinician’s personal judgment and intuition.
- Data Privacy: The system’s effectiveness depends on the collection and analysis of sensitive patient data, raising privacy concerns. (See “Myth #3” below to learn how top Augmented Intelligence vendors like Eleos address this challenge.)
- Adaptability: The system may struggle to adapt to rare or unique cases that deviate from common patterns.
- Initial Resistance: There can be resistance from practitioners due to the perceived complexity of the system. (Check out this blog post for tips on collaborating with providers to foster successful tech implementation.)
How can Augmented Intelligence systematically address major challenges in behavioral health?
Despite the challenges, the potential for Augmented Intelligence to address several pain points in the current behavioral health landscape is significant. It can help manage therapists’ increasing workloads, automate repetitive tasks, and support more informed clinical decision-making.
An article titled, “Pragmatic AI-augmentation in mental healthcare: Key technologies, potential benefits, and real-world challenges and solutions for frontline clinicians,” proposes a framework for pragmatic AI-augmentation. This model outlines automation, engagement, and clinical decision support technologies that can be integrated into everyday clinical practice, paving the way for overcoming some of the challenges facing behavioral health.
- Automation Technologies: Automation streamlines tasks like screening, diagnosis, and recommending treatments. It enables early detection, increases patient access, and improves accuracy. Additionally, automation can handle the bulk of repetitive and cumbersome tasks like documentation so clinicians can focus on the human aspects of care. This, in turn, helps address behavioral health workforce problems like burnout and provider shortages. In essence, automation technologies enhance measurement-based care, optimize healthcare delivery, and reduce administrative burden.
- Engagement Technologies: Engagement tools, such as chatbots and virtual agents, facilitate patient interaction and support. They provide 24/7 availability, particularly in remote areas with limited access to mental health professionals. These technologies can disseminate evidence-based practices, extend therapy beyond in-person sessions, and offer personalized support for homework and skills practice. By augmenting clinician interventions, they enhance the effectiveness and accessibility of behavioral health services.
- Decision Support Technologies: Decision support systems employ machine learning algorithms to make predictions based on large datasets. They enable clinicians to detect mental illnesses earlier and offer tailored treatments. These technologies provide valuable insights, process data efficiently, and integrate new knowledge into models. While they lack human empathy and nuanced judgment, they augment clinicians’ decision-making by identifying effective interventions and improving client outcomes.
Ultimately, these technologies can both enhance care and improve quality of work life for clinicians.
Misunderstandings About AI in Behavioral Health
As Augmented Intelligence gains traction within the behavioral health sector, several misconceptions have emerged that can cloud understanding and incite apprehension. By addressing these myths, we can better appreciate the true capabilities and limitations of AI.
What are some common myths about AI in behavioral health?
Myth #1: AI was Created to Replace Therapists
Contrary to common fears, Augmented Intelligence is not designed to replace therapists. It is a tool specifically created to enhance the capabilities of human behavioral health professionals. The nuanced understanding, empathy, and relational connection a therapist provides are irreplaceable elements of therapy. AI is there to support this process, not to take it over.
Myth #2: AI Understands Everything About a Patient
While AI is incredibly powerful when it comes to pattern recognition and data analysis, it does not possess the depth of understanding a human clinician has. It cannot interpret context, emotions, or the human experience the way a therapist can.
Myth #3: AI Poses Additional Privacy Risks
The use of AI in healthcare raises important privacy and security questions—but when done right, it doesn’t pose any greater risk than existing digital health tools. The key is choosing AI platforms that are actually built for behavioral health and designed with security in mind.
Many generic AI tools, including ChatGPT and other off-the-shelf solutions, aren’t built to meet healthcare compliance and security standards. Some store session data indefinitely, while others rely on third-party cloud services that could introduce privacy risks. OpenAI, for example, has publicly stated that it manually reviews shared data, meaning that any PHI entered into ChatGPT or similar tools could be exposed. What’s more, standard Business Associate Agreements (BAAs) may not fully protect PHI when shared with AI vendors that don’t specialize in healthcare.
That’s why Eleos takes a privacy-first approach to AI. Instead of using third-party models, Eleos built its own in-house AI system trained on de-identified behavioral health data—so PHI is never shared with outside entities. Eleos meets HIPAA and SOC2 security standards and is currently securing ISO 42001 certification, the new global benchmark for AI security. All data stays encrypted—both in transit and at rest—and never leaves secure US-based servers. On top of that, Eleos runs regular security audits and penetration testing to stay ahead of evolving risks.
With the right safeguards in place, even healthcare organizations that handle very sensitive data can use AI confidently without worrying about compliance gaps or security vulnerabilities.
Download our Ultimate Guide to Behavioral Health AI Privacy & Security to get the full scoop on our data security safeguards at Eleos.
Additional Note: Beware of vendors utilizing third-party applications, such as GPT4, as they may share your Protected Health Information (PHI) with other entities. Standard Business Associate Agreements (BAAs) may not cover this, as OpenAI has stated that they manually review shared data. Eleos, conversely, has developed its own AI models in-house—specifically for behavioral health—and does not share PHI with third parties.
Eleos prioritizes data security by adhering to stringent internal processes for data access, using secure physical locations and maintaining high-level certifications (including being HIPAA and SOC2 compliant as well as Deloitte and ISO 27001 & 27799 certified). At Eleos, our data never leaves the US and is always encrypted, both at rest and in transit. We undergo regular penetration testing, continually auditing ourselves and ensuring our vendors meet our rigorous security standards.
Myth #4: AI is Only for Large Practices or Hospitals
AI is scalable and adaptable to various healthcare settings, including individual practices. Regardless of an organization’s size, AI can offer benefits like streamlining administrative tasks and improving patient care.
Myth #5: AI is Difficult to Implement, Integrate, and Use
AI tools are designed to be user-friendly, and many platforms offer training and support to help providers integrate the technology into existing workflows.
Check out our Complete Guide to Behavioral Health AI Implementation for tips from real behavioral health leaders who have successfully adopted AI in their organizations.
Myth #6: AI’s Usefulness is Limited to Data Analysis
While data analysis is a major component of the AI value prop, its impact extends far beyond that. AI fosters more personalized care, enhances treatment planning, reduces clinician burnout by handling administrative tasks, and even assists in predicting and preventing crises through early warning sign identification.
What’s the difference between “dot phrasing” and true AI?
“Dot phrasing” is a common term in the world of electronic health record (EHR) systems, particularly in clinical note-taking tools. This feature uses programmed shortcuts to assist healthcare providers in quickly completing their documentation tasks.
Dot phrasing is basically a digital shorthand system. When a clinician types a period followed by a specific keyword or abbreviation, the system expands it into a more complete phrase or sentence. For example, “.cc” might be automatically replaced with “Chief Complaint.” This method can save significant time when documenting standard procedures or common patient complaints.
However, it’s important to differentiate dot phrasing from true Artificial Intelligence. Dot phrasing might be an intelligent design choice for EHRs, but it doesn’t add any “intelligence” to the provider’s notes. It operates based on predefined templates or rules and doesn’t learn, adapt, or provide personalized insights over time. It’s essentially a reactive system that executes a specific task in response to a specific input.
In contrast, true AI—specifically Augmented Intelligence—adds an extra layer of sophistication. It doesn’t just assist in note-taking; it uses machine learning algorithms to analyze data, draw insights, make predictions, and provide suggestions. It’s a proactive system that continuously learns and improves from the data it processes, offering much more than a mere shortcut for documentation. True AI brings forth the potential to improve care personalization, support decision-making, and enhance the overall quality of healthcare.
The Intersection of AI and Behavioral Healthcare
The intersection of computer processing power and behavioral healthcare operations is ushering in a new era of efficient, personalized, and data-driven care.
How does Eleos leverage AI and Augmented Intelligence technology?
Purpose-built platforms like Eleos leverage AI and Augmented Intelligence technologies to tackle administrative and operational tasks. By leveraging AI systems to handle things like documentation and compliance, behavioral health organizations can significantly reduce the hours their providers spend on non-direct-care activities. This allows care providers to focus more time and energy on delivering high-quality, compassionate care.
Here are some key areas where AI automates and enhances the operational aspects of behavioral health organizations.
Documentation and Record-keeping: AI-powered systems can automate the majority of progress note documentation, ensuring accurate and comprehensive records of patient encounters. By leveraging natural language processing, these systems extract relevant information from clinical conversations and populate notes within electronic health records, reducing documentation time and effort for clinicians.
See how Eleos saved providers at GRAND Mental Health 10 weeks of admin time in just 6 months.
Compliance and Regulatory Requirements: AI can assist in navigating the complex landscape of compliance and regulatory guidelines. It can automatically flag potential errors, inconsistencies, or missing information—helping organizations maintain adherence to required standards and reducing the risk of compliance issues.
Learn how Eleos helps behavioral health organizations review 20x more notes for compliance without adding CDI or CQI staff.
Data Analytics and Reporting: AI enables advanced data analytics capabilities, allowing organizations to derive meaningful insights from vast amounts of behavioral health data. These insights can support evidence-based decision-making, identify trends, assess treatment outcomes, and optimize resource allocation.
By automating these operational tasks, AI and Augmented Intelligence enable behavioral health organizations to streamline workflows, increase efficiency, and enhance overall care delivery. They empower care providers to better engage with patients, make informed decisions, and leverage their clinical expertise to drive improved patient outcomes.
Looking Ahead: The Future of AI and Augmented Intelligence in Behavioral Health
Augmented Intelligence in behavioral health has already been reshaping how clinicians work. Providers are using it to cut down on administrative tasks, streamline documentation, and surface insights that help guide clinical decisions. They’re spending less time on paperwork and more time focused on clients—all with a better understanding of treatment progress over time.
Now, AI is moving beyond documentation support and becoming a more integrated part of care workflows. Instead of simply recording what happens in a session, AI can help therapists recognize patterns across multiple visits, track engagement shifts, and suggest next steps based on treatment history. We’re moving into an era where these tools are seamlessly connected to EHRs, scheduling platforms, and referral networks, reducing the time spent on administrative work.
What’s next is a shift from AI as an assistant to AI as a proactive collaborator. Soon, therapists won’t just review AI-generated summaries; they’ll start sessions with a pre-prepared overview highlighting key themes from past visits, engagement trends, and potential areas for follow-up. Instead of manually scanning notes to track progress, AI will flag subtle shifts and proactively pull research-based recommendations so providers can respond before a client disengages or symptoms escalate.
The more these tools evolve, the more they will allow clinicians to spend their time where it’s needed most: engaging with clients, making informed decisions, and delivering high-quality care without getting lost in the administrative load. These seismic shifts are already happening, and the organizations that embrace technology will be best positioned to deliver high-quality, effective care for decades to come.
Curious how the right AI solution can drive better client engagement and care outcomes in your behavioral health organization? Request a personalized demo of Eleos now.